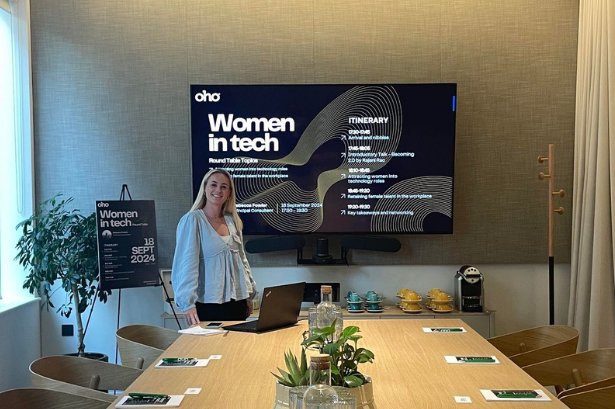
Women In Tech Roundtable- The Future Impact of AI on Software Engineering
20 Mar, 20255 mins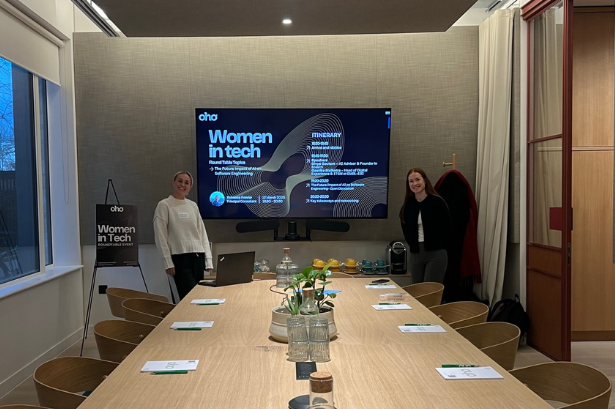
This month we hosted our second Women in Tech Roundtable event, which provided an insightful discussion on how organisations tackle the challenges and opportunities associated with AI adoption. It was a lovely evening, and we got so much out of the conversations, from real-world experiences to thought-provoking insights. Key topics ranged from adopting AI tools like GitHub Copilot, AI ethics policies, licensing concerns, and measuring AI productivity. Here are the key takeaways from the evening, shared by various leaders in the field:
1. AI Adoption in Large vs. Small Organisations
Many leaders in large organisations shared that while AI adoption has been met with enthusiasm in some areas, there are still significant challenges with adopting and implementing in larger, more complex teams. The transition from a complete ban on AI to allowing certain tools, such as GitHub Copilot, has been gradual. However, resistance is often encountered, with developers sometimes bypassing these tools and opting for alternatives like ChatGPT (which comes with its own compliance challenges), especially when they feel it’s more effective.
One key takeaway was that the excitement around AI tools like GitHub Copilot can fade quickly if the technology doesn’t meet expectations around productivity. Many developers initially enthusiastic about using AI for coding found themselves frustrated by the errors AI models often generate, leading to a decrease in productivity rather than improvement. As a result, organisations must carefully consider the contexts in which these tools will be used and align expectations with real-world outcomes.
In contrast, startups and smaller companies tend to adopt AI tools more rapidly and with fewer restrictions. With leaner teams and a greater need for efficiency, startups are more willing to experiment, integrating AI into their workflows at an accelerated pace. They often use AI as an experimental tool, particularly in the prototyping stages, to rapidly test ideas and refine their products. The lack of rigid policies allows for quicker decision-making, and developers often have more autonomy in choosing the AI tools that best suit their needs. However, this flexibility comes with its own challenges; smaller companies may struggle with AI governance, security concerns, and ensuring that AI-driven decisions align with business objectives.
Ultimately, while large organisations face resistance due to complex structures and compliance concerns, startups benefit from agility but must remain mindful of responsible AI implementation. Finding the right balance between innovation and oversight is crucial, regardless of company size.
2. The Challenge of Licensing and Intellectual Property
A major concern for many organisations is managing intellectual property (IP) when using AI tools. In particular, the licensing implications of code generated by AI models, especially when tools like GitHub Copilot and ChatGPT are involved, are complex. The worry arises when proprietary code or sensitive information is used by AI, potentially creating IP risks or conflicts. But it is not just about what employees input into ChatGPT, such as confidential company code or data. It is also about the uncertainty of what ChatGPT might extract or infer from it. Since AI models generate responses based on vast datasets, there is always a risk that outputs may inadvertently incorporate proprietary patterns, structures, or even fragments of sensitive information. This highlights the importance of establishing clear AI ethics policies and guidelines that govern the use of AI, ensuring that any proprietary information stays secure.
One suggestion shared was to define a specific “AI ethics policy” that regulates how AI is used in the development process, especially when it comes to sensitive data or projects with high IP value. In one case, a company had to weigh the pros and cons of AI tools like GitHub Copilot, balancing developer productivity with the potential risks of proprietary information leakage.
3. Managing Developer Productivity and Expectations
The impact of AI on developer productivity is not straightforward. While some have reported a 20-30% productivity boost from using AI tools, others have seen minimal improvements or even setbacks due to AI inaccuracies. Factors such as understanding the complexity and the context of the code are often a limitation for AI, therefore human interference in the AI loop is often necessary.
For example, one participant noted that teams working on legacy systems or technical debt had seen significant productivity gains, while those working on newer or more complex codebases were less impressed. It’s crucial to note that AI is still in the experimental phase for many organisations. Developers must be able to experiment with different tools and workflows without expecting immediate and uniform results. There is an expectation that AI tools will start to support more complex codebases but there will always be a need for human interaction in the AI Loop.
4. Training and Cultivating a Strong AI Culture
Several speakers stressed the importance of training their developers but noted that building a culture of responsible AI use is even more crucial. Training programs should not be treated as tick-box exercises but rather as part of an ongoing effort to instil a deep understanding of AI tools and their limitations. The culture of AI adoption within an organisation should be driven from the top down, with leadership continuously reinforcing ethical practices and encouraging open dialogue about the pros and cons of using AI.
Some organisations initially implemented a “zero-trust” policy, especially regarding proprietary or sensitive data. However, as AI training expanded and employees became more aware of the rules for handling highly confidential work, greater autonomy was introduced. This approach balances data security with the benefits of using AI tools to enhance productivity.
5. Metrics for AI Success: Beyond Traditional Tracking
When it comes to measuring AI success, organisations are grappling with how to track and validate improvements. Traditional metrics, such as the number of check-ins or churn rate, do not adequately capture the nuanced benefits of AI. Leaders are beginning to recognise that AI’s impact on productivity must be assessed in a more context-specific way, focusing on the business value of AI-driven solutions rather than just individual developer performance.
One example shared was the shift towards measuring the time it takes to move from ideation to product development, particularly in prototyping teams. These teams, which are focused on getting features to market quickly saw the highest productivity spikes when using AI tools. Metrics tied to faster release cycles and the introduction of new, differentiating features were more meaningful for the business than traditional developer productivity measurements.
6. Ensuring AI is Used Appropriately
Managing how developers use AI tools remains a challenge, especially when tools like ChatGPT are freely accessible. The question arises: How do organisations prevent developers from using unapproved AI platforms or from inadvertently exposing sensitive data to external AI models?
One speaker shared their experience with setting up internal LLMs (large language models) for organisation-specific tasks. This strategy not only provided a controlled environment for AI usage but also addressed concerns about using external models that could potentially compromise sensitive information.
Conclusion: The Road Ahead for AI
The key takeaway from the Women in Tech Roundtable event is that AI adoption in organisations is still in its early stages, and organisations must approach it thoughtfully. There is no one-size-fits-all solution. Companies must carefully consider the types of projects, the level of AI maturity, and the context in which AI tools will be applied.
Successful AI adoption hinges on clear ethical guidelines, proper training, cultivating a strong AI culture and setting realistic expectations. The pace at which AI technologies evolve means that organisations need to remain flexible, continuously assessing the tools they adopt and the metrics they use to measure success. Above all, building a culture that values responsible AI usage while encouraging innovation will be key to achieving long-term success with AI in the workplace.
As for the question of whether AI will make jobs redundant, the answer is no. Companies won’t be eliminating roles; rather, they will need to learn how to adapt, upskill their workforce, and find new ways to leverage AI tools to enhance productivity. The future is about collaboration between humans and AI, not replacement.
We're planning to host more Round Table Events soon! If you're interested in joining, please register your interest here: https://ow.ly/z5Et50TqI3G
Or get in contact with Rebecca at rebeccaf@oho.co.uk